Lack of synthetic data generation datasets is often cited as the major development obstacle for deep learning systems. Creating and labeling sufficient data from physical testing and other non-algorithmic methods, such as photography, can be extremely time consuming or impossible. The problem is further compounded when the product or process being studied is under development and no physical data exists, or if the items of interest are rare and underrepresented in the physical dataset.
We create data sets using a variety of proprietary synthetic data generation methods which virtually eliminates machine learning development obstructions. The synthetic data sets can be multi-class and are developed for both regression and classification problems. Data annotation is automatic, zero cost, and 100% accurate. Our synthetic data generation data set are provided using a database and labeling schema designed for your requirements. Contact us to discuss your particular machine learning data needs.
Learn more about synthetic data at Wikipedia.
Synthetic Data Generation Used for Retail Merchandising Audit System
In this example created by Deep Vision Data, a deep learning model based on the ResNet101 architecture was trained to classify product SKU’s, stock outs and mis-merchandised products for a retail store merchandising audit system. The model was trained with 20,000 synthetic product images using a 50-50 split of structured and unstructured domain randomized subsets and an 80-20 training/validation data split. Model validation was also completely done with 100% synthetic training data. The test set was comprised of actual photos; a sampling of results images are shown to the right.
Domain randomization (DR) is a powerful tool available with synthetic data: it enables the creation of data variability that encompasses both expected and unexpected real-world input, forcing the model to focus on the data features most important to the problem understanding. DR is much more costly and difficult to implement with physical data. For example, the creation of a dataset of thousands of products where each product is shown in thousands of poses on dozens of backgrounds requires many millions of labeled images. That dataset is easily generated synthetically, while virtually impossible to create using physical product photos. In addition, the distribution of rare (but possibly very important) events or conditions is easily controlled, unlike physical data where rare occurrences are by definition poorly represented.
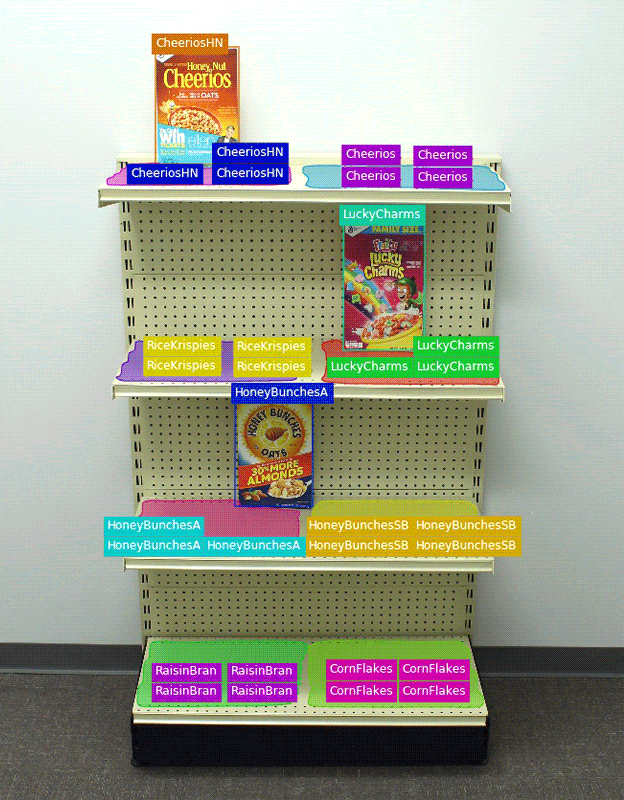
Synthetic training data can be utilized for almost any machine learning application, either to augment a physical dataset or completely replace it. By effectively utilizing domain randomization the model interprets synthetic data as just part of the DR and it becomes indistinguishable from the physical information. Synthetic data generation is inherently less costly, faster to create, perfectly annotated, and isn’t constrained by availability, time or even the physics of the natural world.
Synthetic Data Generation Methods
Our methodology for synthetic data generation is a matrix of multiple input sources and software systems. The model is populated with real or simulated data from CAD/engineering simulations, computer graphics code, real-time simulator data, industrial computed tomography scanning, and custom software applications. Our data scientists and engineers are able to use the data capture to create multi-million synthetic data sets with full annotation utilizing our state-of-the-art NVIDIA AI Server. To help automate the data creation process, Kinetic Vision has developed AiVision™ Generate – a proprietary software system that creates data sets with full variability control including randomization, exposure compensation, and scene parameter controls. For more information watch this video on AiVision™ Generate.
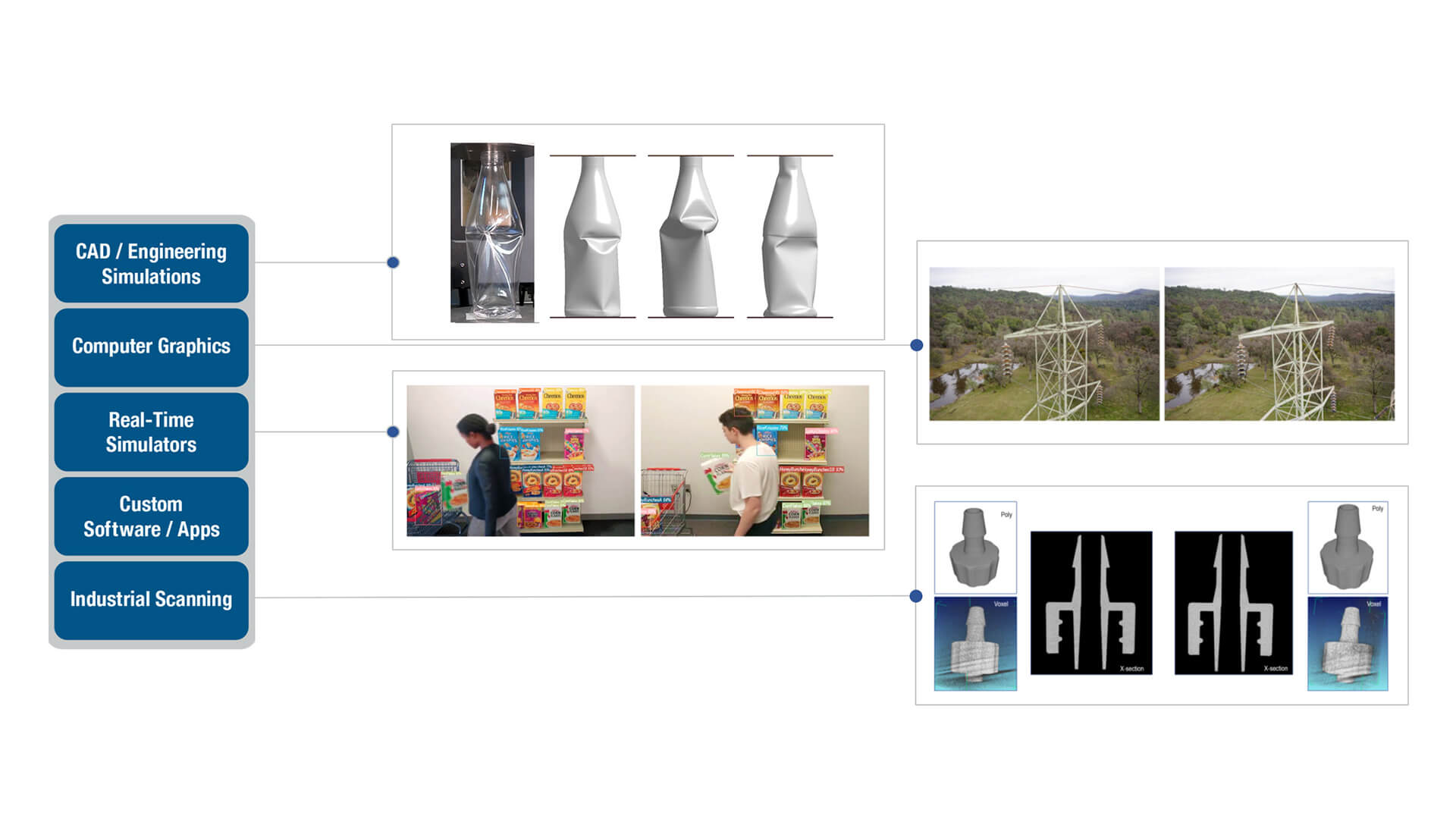
Product Images
Synthetic product images are 100% virtual and can include variability in pose, lighting, material finish and many other factors. Portions of the images can be occluded to simulate handling or other situations, and the renderings can be photorealistic, grey-scale or silhouette. Need a million images in a few days? No problem!
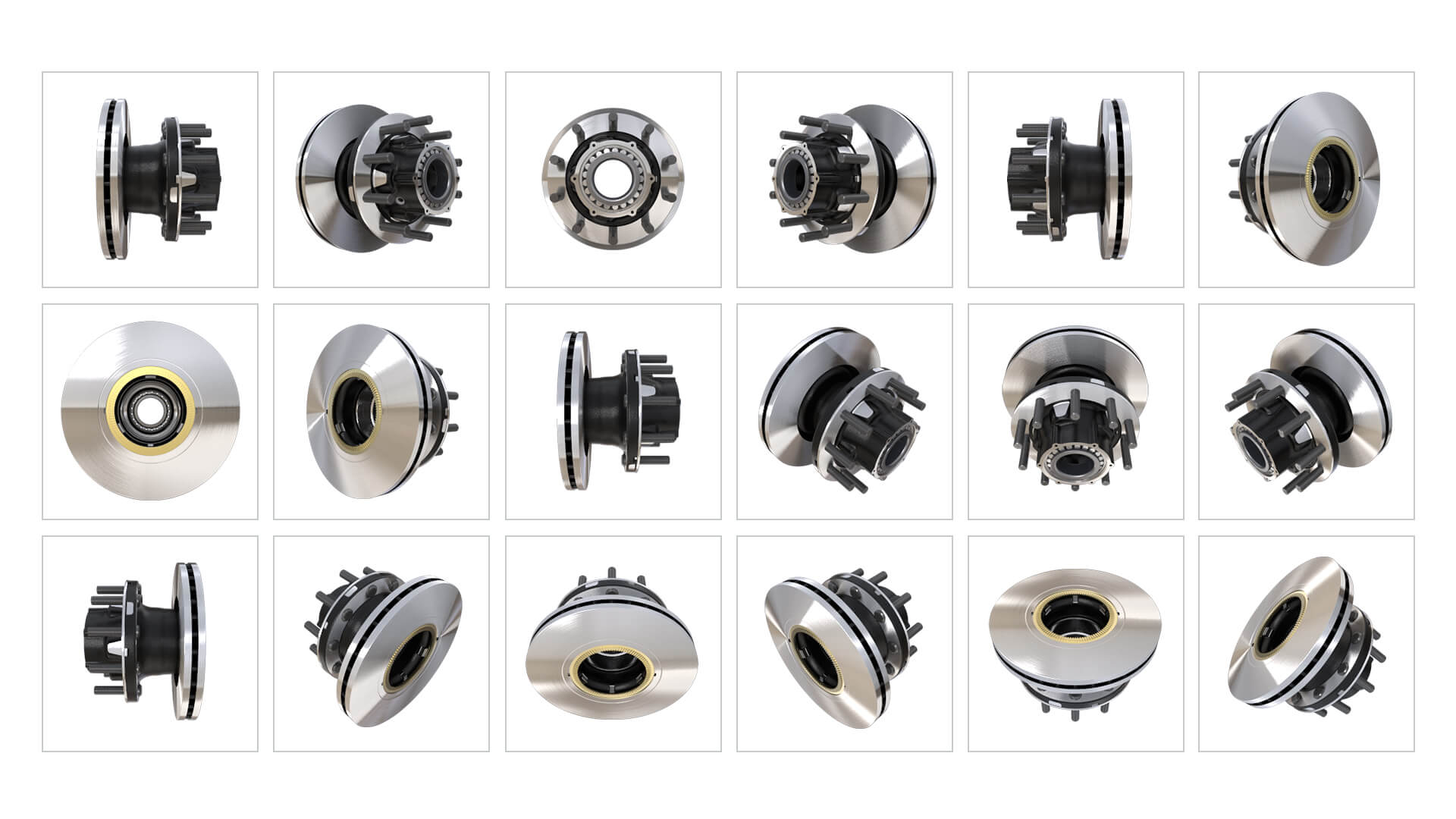
Hybrid Images
Need thousands of training images of your product presented in a specific physical environment, and need them fast? Mobile devices running augmented reality (AR) apps can be utilized to quickly create hybrid images of virtual objects in physical environments. Useful for when the environment contains important class information or for domain randomization.
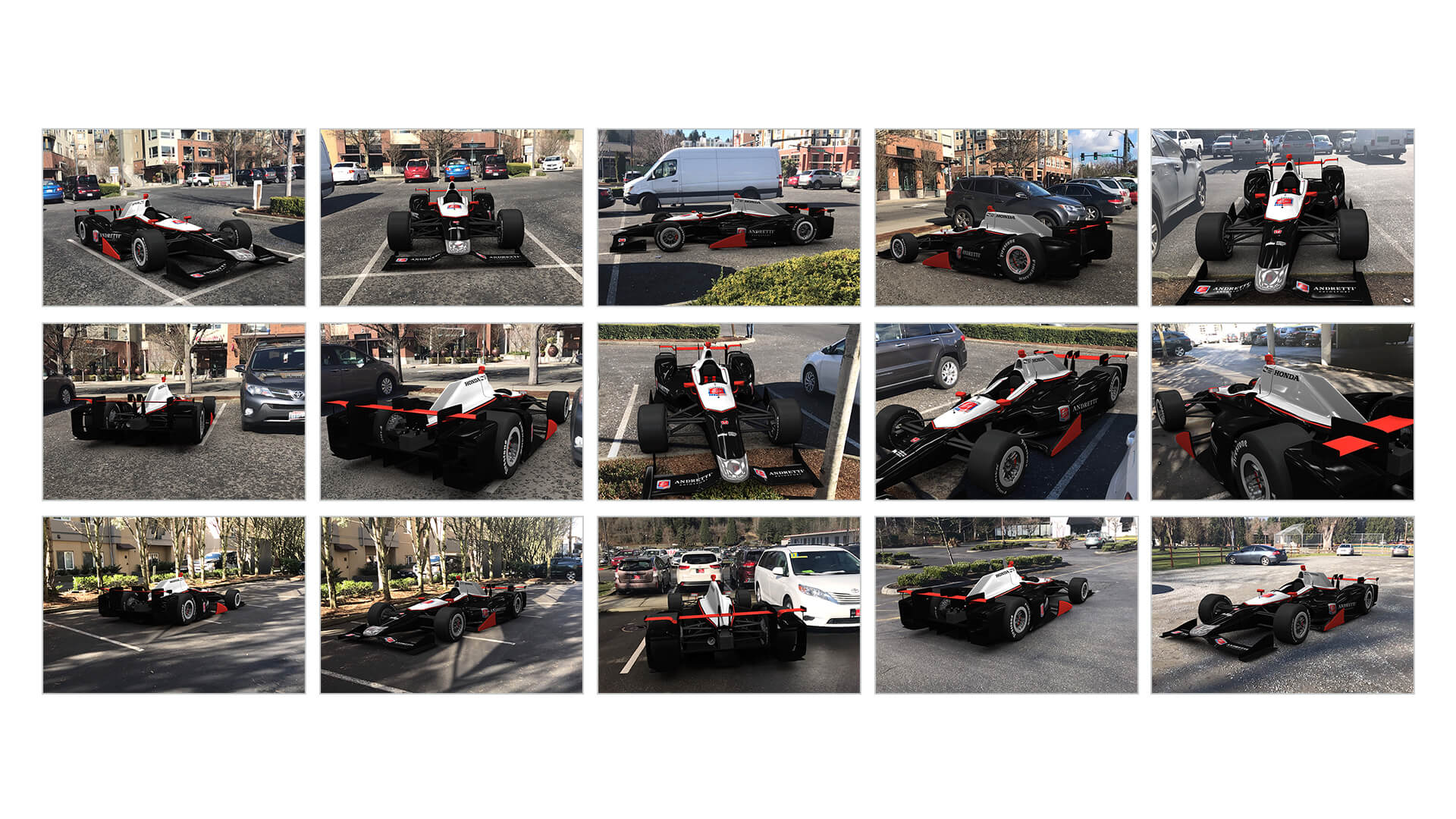
People and Environments
Synthetic environment images can be interior or exterior and include people, plants/trees and any inanimate object. Used to train systems to identify safety, compliance, stocking, manufacturing process deviations, traffic incidents, human behavior and many other functions. Scenes are database-driven to enable rapid configuration variation.
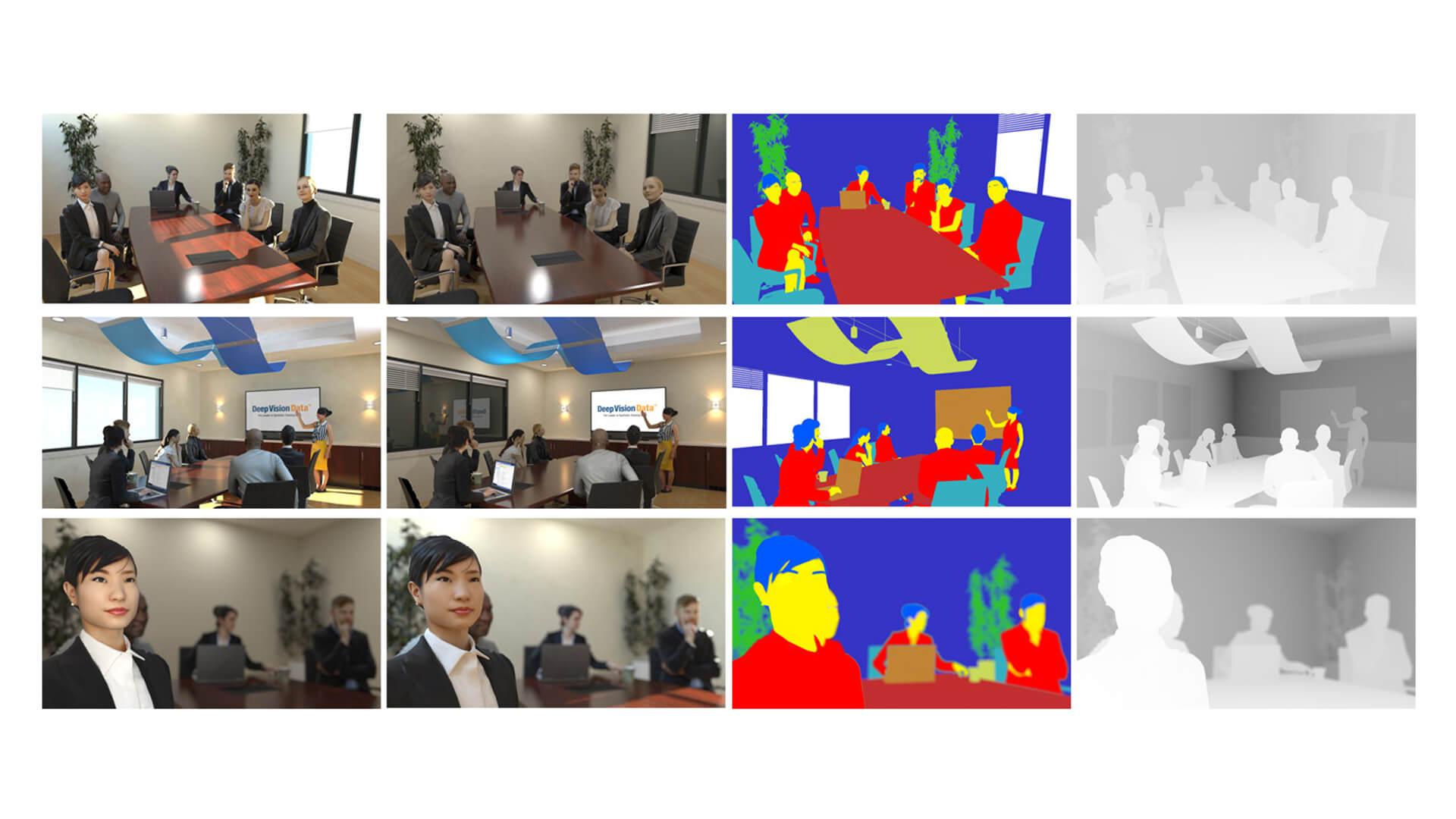
Real-Time XR Environments
Real-time simulators utilize game engines and custom software to replicate physical products, systems and processes. These Digital Twins are implemented in Extended Reality (XR) environments to create fully immersive training experiences. Multiple simultaneous instances of the real-time simulation environment can be utilized to rapidly speed up the training process.
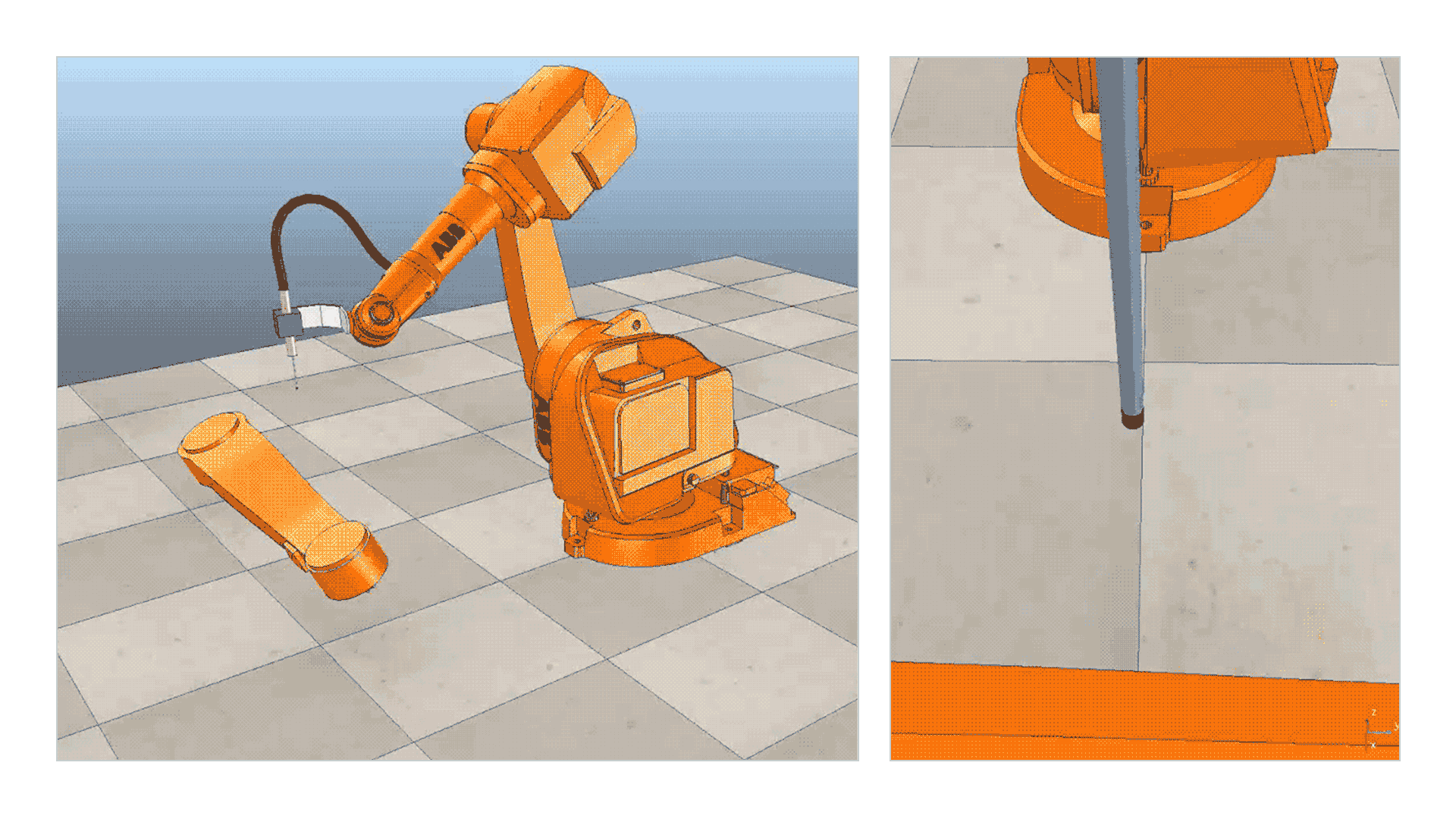
Modeling and Simulation
Modeling and simulation is used to explore product life cycle, performance, etc. due to variation in component dimensions, production quality, process parameters and other real-world variances. This data can be used to create intelligent CADTM systems that guide users to design products or systems which have optimized cost, performance or weight goals.
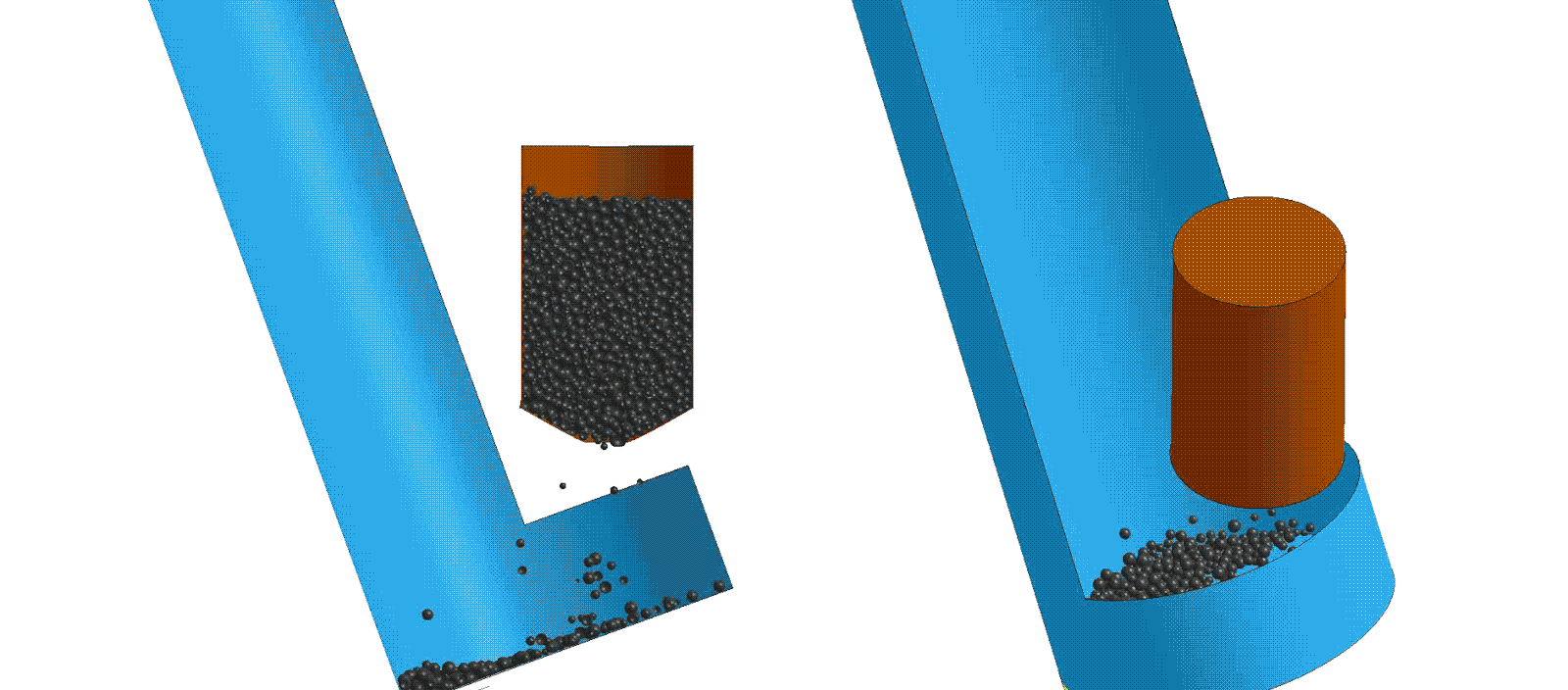
Design Variation
Computer-aided design (CAD) tools are used to algorithmically vary component dimensions or assembly parameters. The resulting data can be utilized to train intelligent production vision systems to identify manufacturing or quality issues that are too complex for traditional in-line processes. Coupled with industrial 3D scanning, machine learning creates the next evolution of part inspection.
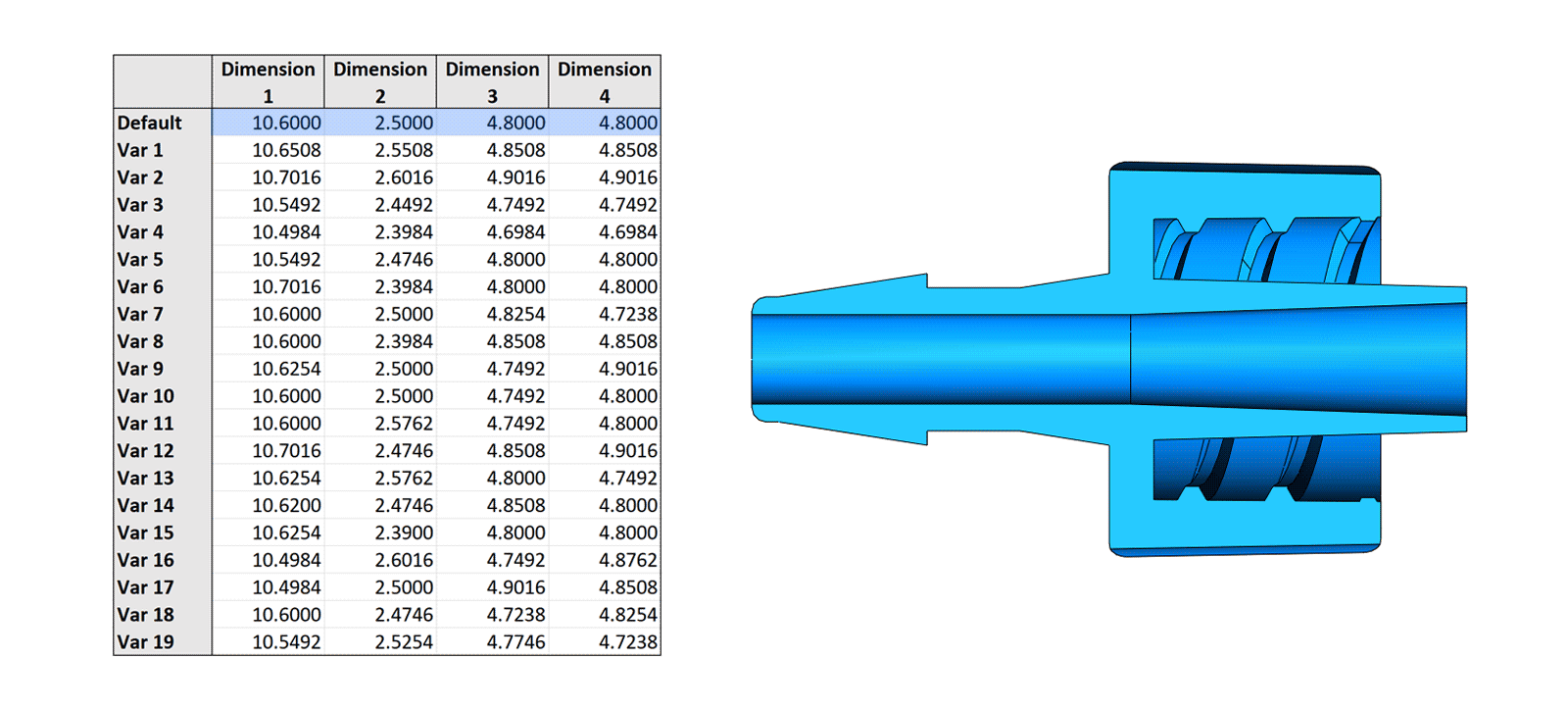