Surrogate Modeling helps designers and engineers have a better understanding of the dimensional sensitivity of their design. Using a Design of Experiments (DOE) statistical approach the relationship between user inputs and performance outputs are determined by running a number of simulations. The resulting model is used to quickly test and optimize the product performance by adjusting the input parameters and their expected variance. Often these input parameters are part dimensions and tolerances, but other variables can be considered such as material properties, material cost, component loading and manufacturing parameters.
The power of surrogate modeling is that once you generate a surrogate model you minimize the need to run additional simulations for other part designs since you already fully understand performance of all variable interactions. This approach allows design professionals the ability to tune product performance while minimizing overall cost.
L-Bracket Example
In this simplified example a metal L-bracket is optimized for minimum part cost considering simultaneous vertical and horizontal loading. The design variables are the bracket material thickness and width. Even a simple illustrative problem like this requires more than a dozen FEA simulations to manually optimize the design. Manual optimization of real-world engineering problems might require hundreds or even thousands of simulation computer runs, and the result still might still be erroneous as local minimums (apparent optimal designs) may occur. Surrogate modeling greatly reduces the time and compute resources required to solve these complex problems, and visual inspection of the resulting response surfaces helps prevent selection of non-optimal design solutions.
See also Product Design + Development.
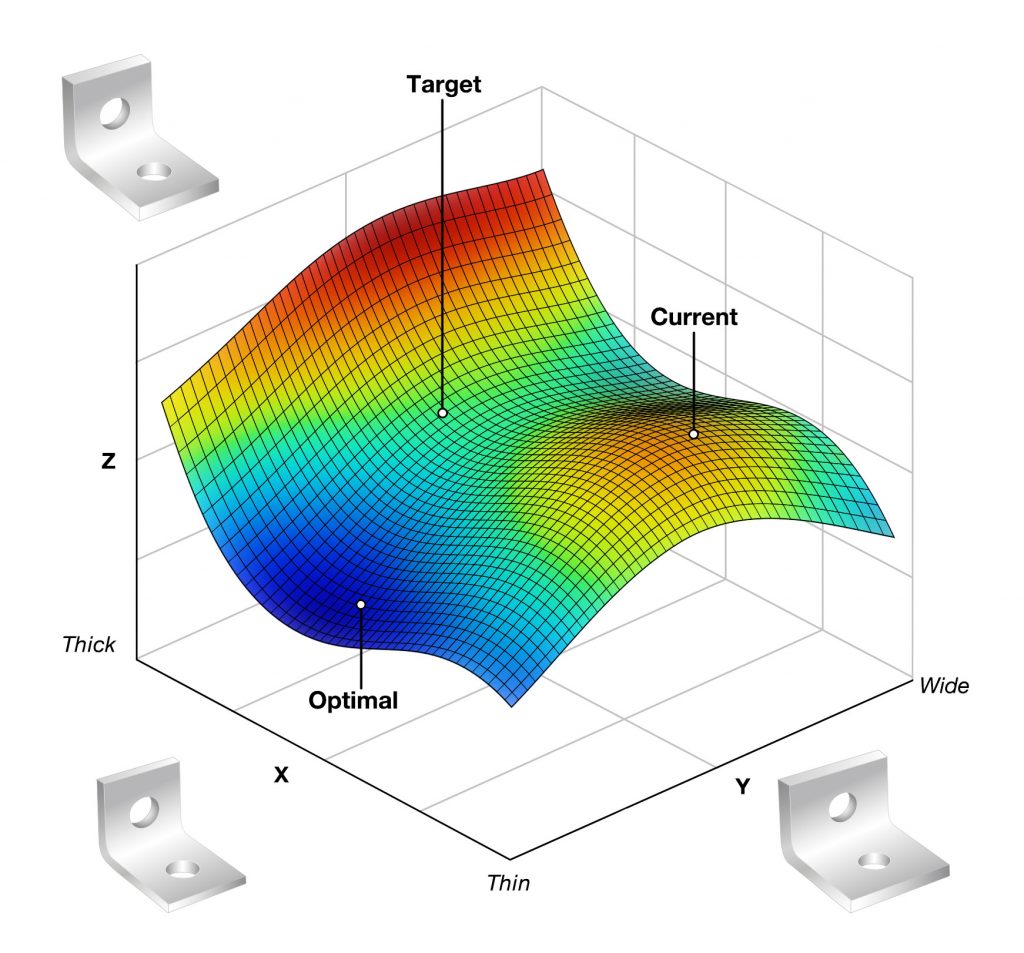